Recommender systems can explore individual demands of users by analyzing their behavior. In this way, long-tail items will be individually recommended to the appropriate users, and thus recommender systems help users find the items, which they want but cannot find.
ILEARN aims to resolve the related problems in the practical application scenario of recommender systems. So far, our proposed works cover commodity recommendation of e-commerce and micro-video personalized hashtag recommendation of multi-media.
To solve the efficiency and the cold start problem of commodity recommendation, we combined deep learning with typical recommendation algorithms. We have elaborated discrete factorization machines for fast feature-based recommendation and attribute-to-feature adversarial learning for new-item recommendation.
The hashtag is the most representative information obtained by abstracting and summarizing micro-videos,which represents user’s personal understanding and preference of the content. However, a large number of micro-videos have no hashtags or hashtags are inaccurate or incomplete, so an automated micro-video tagging system has a great application value. We proposed a multi-view representation interactive embedding model based on graph structure for information propagation, which is used for micro-video personalized hashtag recommendation. We use Graph Convolutional Neural Network to guide the information propagation and mine the structure and semantic relationships of hashtags to solve the long tail distribution problem.
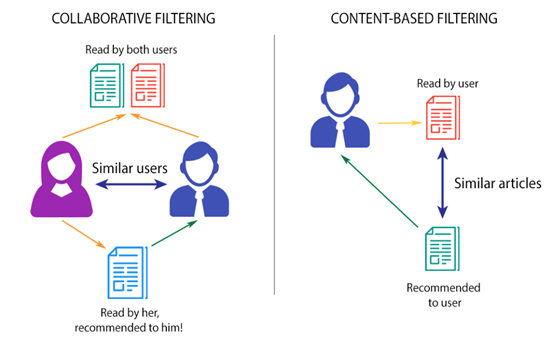