何向南
简介:Dr. Xiangnan He is currently a postdoctoral research fellow with the Lab for Media Search, National University of Singapore. His research interests span recommender system, information retrieval, multi-media and natural language processing. He has over 20 publications appeared in several top-tier conferences such as SIGIR, WWW, MM, CIKM, IJCAI and AAAI, and top-tier journals including TKDE and TOIS. His work on recommender system has received the Best Paper Award Honorable Mention of ACM SIGIR 2016. Moreover, he has served as the PC member for the prestigious conferences including SIGIR, WWW, MM, CIKM and EMNLP, the Co-chair of CIKM 2017 workshop on “Social Media Analytics for Smart Cities”, and the invited reviewer for prestigious journals including TKDE, WWWJ and TIIS.
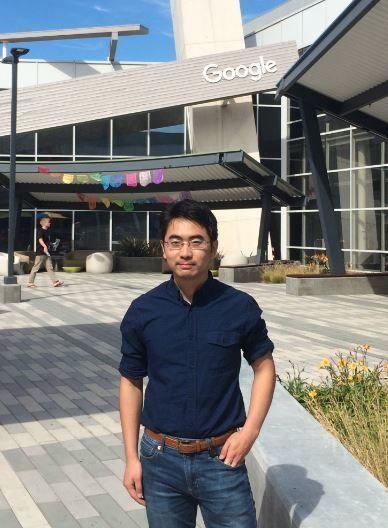
报告题目:Neural Collaborative Filtering
摘要:In recent years, deep neural networks have yielded immense success on speech recognition, computer vision and natural language processing. However, the exploration of deep neural networks on recommender systems has received relatively less scrutiny. In this work, we strive to develop techniques based on neural networks to tackle the key problem in recommendation — collaborative filtering — on the basis of implicit feedback. Although some recent work has employed deep learning for recommendation, they primarily used it to model auxiliary information, such as textual descriptions of items and acoustic features of musics. When it comes to model the key factor in collaborative fltering — the interaction between user and item features, they still resorted to matrix factorization and applied an inner product on the latent features of users and items. By replacing the inner product with a neural architecture that can learn an arbitrary function from data, we present a general framework named NCF, short for Neural network-based Collaborative Filtering. NCF is generic and can express and generalize matrix factorization under its framework. To supercharge NCF modelling with non-linearities, we propose to leverage a multi-layer perceptron to learn the user-item interaction function. Extensive experiments on two real-world datasets show significant improvements of our proposed NCF framework over the state-of-the-art methods. Empirical evidence shows that using deeper layers of neural networks offers better recommendation performance.
简介:Dr. Zhiyong Cheng currently is a postdoctoral research fellow with School of Computing, National University of Singapore. He received the Ph.D. degree from Singapore Management University in 2016. His main research interests include multimedia search and recommendation, information retrieval and social media analysis, with specific focus on mining knowledge from social media to facilitate user-centered information retrieval & recommendation. He has authored and coauthored papers that have been published in top-tier conferences and journals, such as SIGIR, IJCAI, ICMR, TOIS, and TKDE.
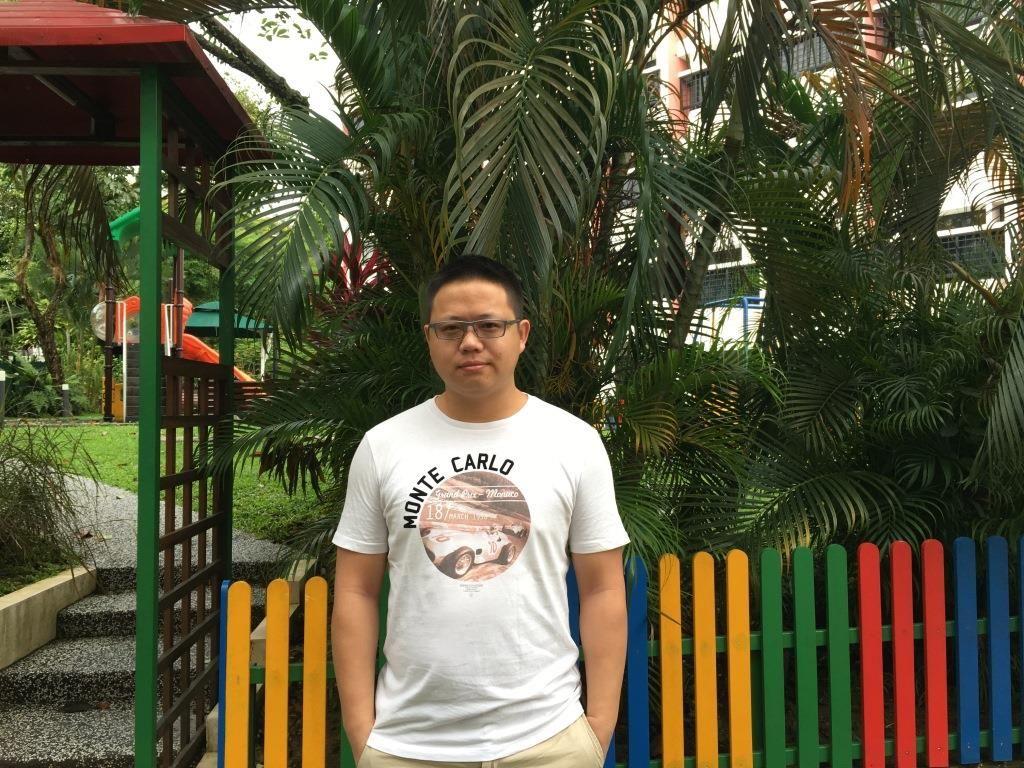
报告题目:User-aware Music Retrieval via the Exploration of Online User Behaviors
摘要:Rapid advances in mobile devices and cloud-based music streaming services have brought about a fundamental change in the way people consume music. Mobile devices become the mainstream platforms allowing people to enjoy favorite music anytime and anywhere. User’s music preferences are influenced by user’s music tastes and local contexts, such as environment factors (e.g., location) and user states (e.g., activity and mood). As mobile devices are designed for personal media consumption and usually used on the move, it is important to consider user’s personal music tastes and the local contextual factors in music retrieval and recommendation. I will introduce our recent two works of user-aware music retrieval. When interacting with the social music portals (e.g., Last.fm), users leave rich digital footprints, which contain the details of personal music listening history. By analyzing users’ online listening behaviors and social tags, we study the problem of user-aware text-based music retrieval, which takes users’ music preferences on songs into account. In the first work [Cheng et al. SIGIR 2016], we propose novel Dual-Layer Music Preference Topic Model (DL-MPTM) is proposed to construct latent music interest space and characterize the correlations among (user, song, term). Based on the DL-MPTM, we further develop an effective personalized music retrieval system. As the personalized retrieval systems require sufficient user’s personal listening logs, and thus suffer from the cold-start problem. Therefore, in our second work [Cheng et al. SIGIR 2017], we exploit users’ demographic information (e.g., gender, age, and country), which are relatively easy to obtain in personal platforms (e.g., mobile phones), to relieve the cold-start problem. It is well-known that user specific information has fundamental influence on personal music tastes. To capture the influence of those factors in retrieval, we propose a User-Information-Aware Music Interest Topic (UIA-MIT) model to associate user’s music preference, song, and semantic tags in a latent music interest space, which can facilitate music retrieval and re-ranking. To validate the effectiveness of the proposed systems, we construct several large-scale music datasets and conduct experiments to compare these systems with other competitors. The experimental results demonstrate the superiority of our systems in terms of search accuracy. We hope our works can shed light on the direction of developing user-centric music retrieval systems and motivate more researches on this area.
魏巍
简介:男,1983年生,博士,华中科技大学计算机学院副教授。2012年博士毕业于华中科技大学计算机学院。在博士及博士后期间先后在微软亚洲研究院 (MSRA),新加坡南洋理工大学(NTU)、新加坡国立大学(NUS)及新加坡管理大学(SMU)从事科学研究工作。主要研究领域包括信息检索、数据挖掘(文本挖掘/社交媒体分析与挖掘)、自然语言处理、机器学习、推荐系统等。在相关领域已发表高水平学术会议及期刊论文近二十篇, 如AAAI, SIGKDD, CIKM, IEEE TKDE, IEEE TSMC-B, IEEE TC, ACM TWEB等。 Dr. Wei Wei is Associate Professor with the School of Computer Science and Technology at Huazhong University of Science and Technology (HUST). He received his PhD degree in Computer Science from HUST in 2012. His research domains include Information Retrieval, Text Mining, Natural Language Processing, Machine Learning and Recommender Systems. He has published nearly 20 papers in the highly referred journals and conferences such as AAAI, SIGKDD, CIKM, IEEE TKDE, IEEE TSMC-B, IEEE TC, ACM TWEB.
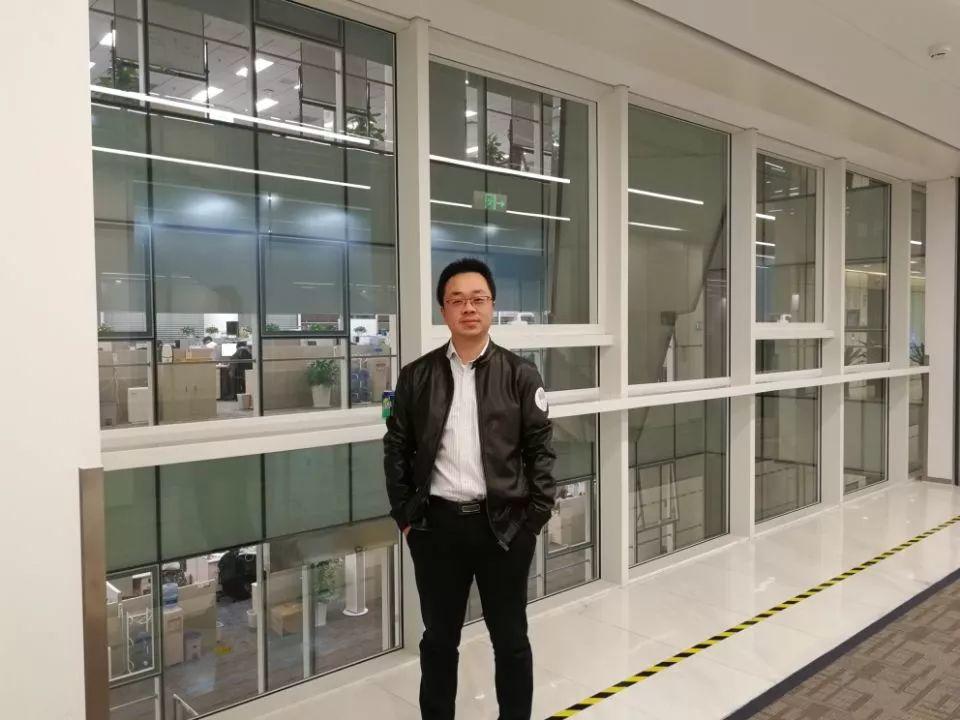
报告题目:Learning to Find Topic Experts
摘要:Expert finding (a.k.a., expert search) aims at identifying people with the relevant expertise or experiences on a given topic query,has been studied broadly in domains such as enterprise,question answering, Web, social network and academic society. Recently, expert finding has become a hot topic along with the flourishing of social networks such as micro-blogging services like Twitter. Finding experts in Twitter is an important problem because tweets from experts are valuable sources that carry rich information (e.g., trends) in various domains. In this talk, we will present an overview of expert finding in different domains, and summarize different algorithms or models in such domains based on different domain knowledge. Then, we will mainly focus on introducing the work in the domain of social network, previous methods cannot be directly applied to Twitter expert finding problem, since they generally relies on the assumption that all the documents associated with the candidate experts contain tacit knowledge related to the expertise of individuals, which might not be true in Twitter. In addition, several attempts use the relations among users and Twitter Lists for expert finding. Nevertheless, these approaches only partially utilize such relations. To this end, we develop a probabilistic method to jointly exploit three types of relations (i.e., follower relation, user-list relation, and list-list relation) for finding experts. Finally, we will discuss the potential future directions in this domain.
任昭春
简介:任昭春博士目前在京东数据科学实验室担任研究科学家。在这之前他在伦敦大学学院(University College London)担任博士后研究员(Research Associate),并于2012年和2014分别在德国马克斯普朗克信息所(D5,MPII)和美国哥伦比亚大学短期访问。任昭春2016年在荷兰阿姆斯特丹大学取得博士学位。他的研究方向为信息检索,文本挖掘和社交媒体的内容分析。自2011年起,他在信息检索领域顶级会议和期刊上发表30余篇论文。除此之外,任昭春还多次担任信息检索领域相关会议和期刊的编辑和组织审稿工作。
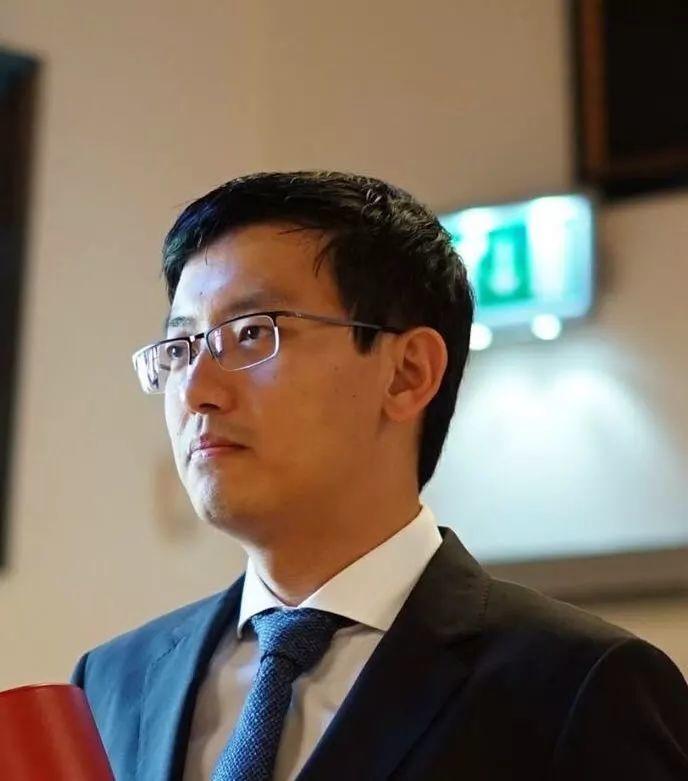
报告题目:Social Media Summarization: From Content to Viewpoints
摘要:A key characteristic of social media research is the ambition to monitor the content of social media, i.e., text from social media platforms, social relations among users, and changes in social media data over time. Our research focuses on understanding social media by extracting salient information from social media. Our first line of work is the summarization of microblogging posts, based on a user’s history and collaborative influences from “social circles.” Our second line of work is the summarization of answers in non-factoid CQA, where the shortness, sparsity and diversity of answers form interesting challenges for summarization. Our third line of work is the summarization of viewpoints on social media. Given a set of opinionated documents, we address the task of summarizing contrastive themes by selecting meaningful sentences to represent contrastive themes in those documents; thereafter, we address the viewpoint summarization of multilingual streaming corpora, by monitoring viewpoints for a running topic and selecting a small set of informative documents.
王翔
简介:Xiang Wang is currently a Ph.D. student in the School of Computing, National University of Singapore. He received the B.E. degree in computer science and engineering from Beihang University, Beijing, in 2014. His research interests include information retrieval, social media analytics, recommender system, and user profiling. Various parts of his work have been published in top forums, such as SIGIR, TOIS, and MM. He has served as reviewers and sub-reviewers for various conferences, such as MMM, ICMR, WebScience, and SIGIR.
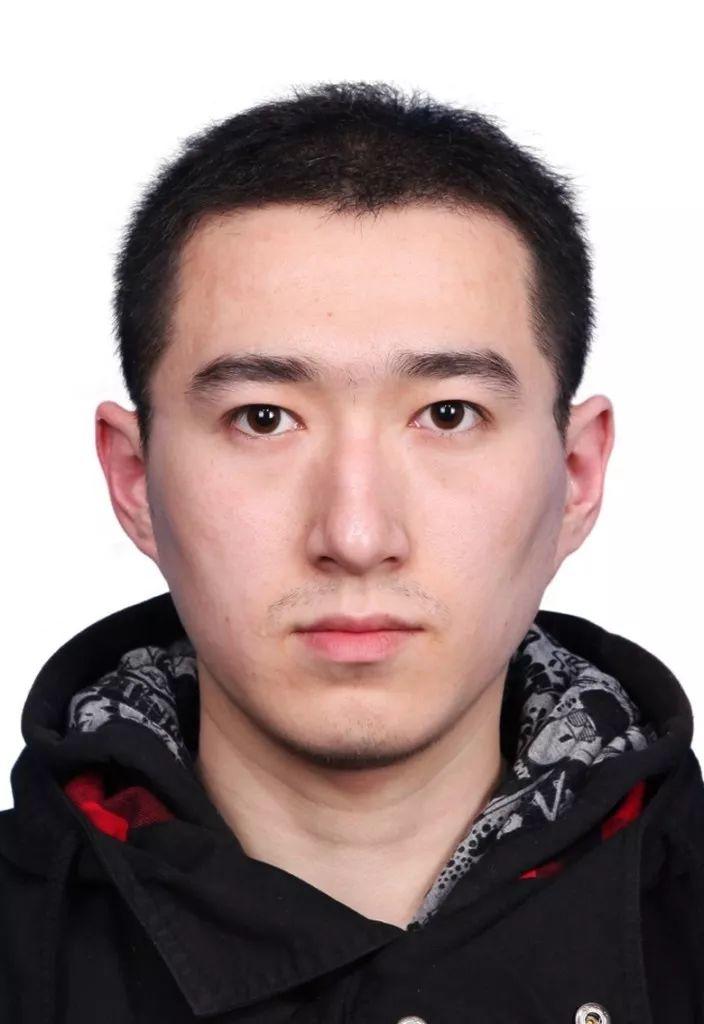
报告题目:Item Silk Road: Recommending Items from Information Domains to Social Users
摘要:Online platforms can be divided into information- and social-oriented domains. The former refers to forums or Ecommerce sites that emphasize user-item interactions, like TripAdvisor and Amazon; whereas the latter refers to social networking services (SNSs) that have rich user-user connections, such as Facebook and Twitter. Despite heterogeneity, these two domains can be bridged by a few overlapping users, dubbed as bridge users. In this work, we address the problem of cross-domain social recommendation, i.e., recommending relevant items of information domains to potential users of social networks. To our knowledge, this is a new problem that has rarely been studied before. Existing cross-domain recommender systems are unsuitable for this task since they either focus on homogeneous information domains or assume that users are fully overlapped. Towards this end, we present a novel Neural Social Collaborative Ranking (NSCR) approach, which seamlessly sews up the user-item interactions in information domains and user-user connections in SNSs. In the information domain part, the attributes of users and items are leveraged to strengthen the embedding learning of users and items. In the SNS part, the embeddings of bridge users are propagated to learn the embeddings of other non-bridge users. Extensive experiments on two real-world datasets demonstrate the effectiveness and rationality of our NSCR method.
张含望
简介:Dr. Hanwang Zhang is currently a research scientist at the Department of Computer Science, Columbia University, USA. He has received the B.Eng (Hons.) degree in computer science from Zhejiang University, Hangzhou, China, in 2009, and the Ph.D. degree in computer science from the National University of Singapore in 2014. His research interest includes computer vision, multimedia, and social media. Dr. Zhang is the recipient of the Best Demo runner-up award in ACM MM 2012, the Best Student Paper award in ACM MM 2013, and the Best Paper Honorable Mention in ACM SIGIR 2016. He is also the winner of Best Ph.D. Thesis Award of School of Computing, National University of Singapore, 2014. Dr. Zhang serves as an associate editor in Neurocomputing and MTAP, and reviewers in various journals and conferences such as CVPR, MM, TIP, TMM, TOMCCAP, TCSVT, and Neurocomputation.
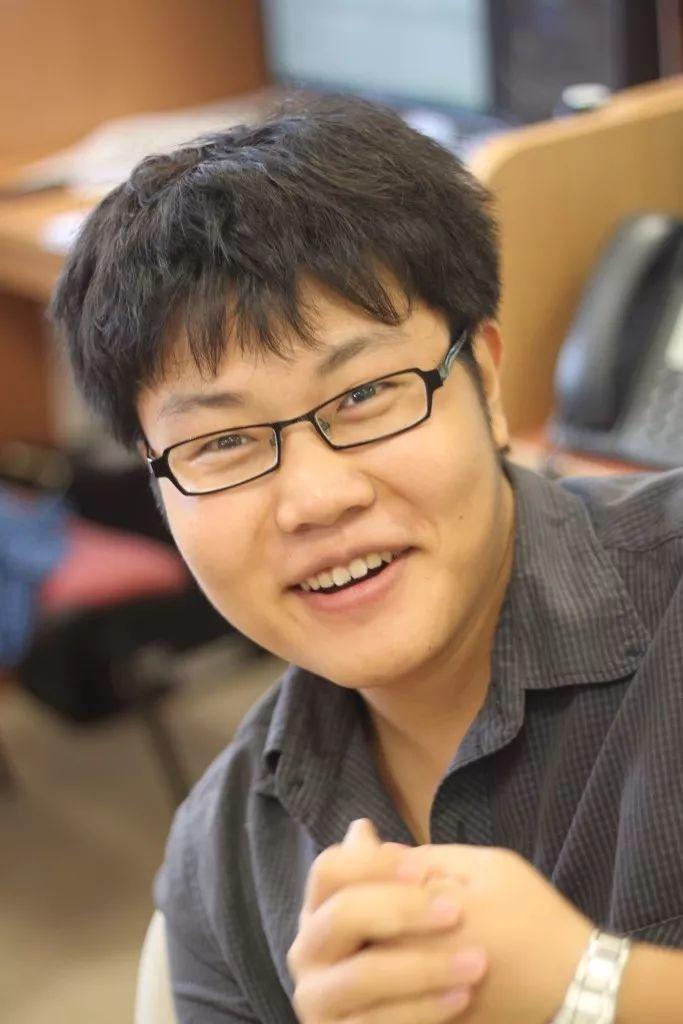
报告题目:From Object Detection to Relation Detection
摘要:I will go through the novel visual detection task: Visual Relation Detection in this group meeting. Visual relations such as “person ride bike” and “bike next to car”, offer a comprehensive scene understanding of an image, and have already shown their great utility in connecting computer vision and natural language. I will first cover supervised VRD using object bounding boxes and then introduce my recent work about Weakly Supervised VRD (WSVRD) with annotations only at the image-level. For supervised VRD, the main technical challenge is how to model the large visual diversity of predicate, say, “riding’’ can be observed in many visual relations such as “person-ride-horse’’, and “person-ride-bike’’. We propose a Visual Translation Embedding model to reduce the predicate learning to a translation vector learning in the relation embedding space. For WSVRD, the challenge is that the absence of instance-level annotation (i.e., bounding box) result in combinatorial search of pairwise object proposals, e.g., for 1000 proposals, we would search for 1,000,000 pairs for potential relations. To this end, we propose a pairwise and parallel region-based fully-convolutional network (PPR-FCN) architecture. We will see how to design the pooling strategy for relation learning.
宋井宽
简介:Jingkuan Song is currently a Research Scientist in Columbia University. He joined University of Trento as a Research Fellow sponsored by Prof. Nicu Sebe (2014-2016). He obtained his PhD degree in Information Technology from The University of Queensland (UQ), Australia, in 2014. He received his BS degree in Computer Science from University of Electronic Science and Technology of China. His research interest includes large-scale multimedia retrieval, image/video segmentation and image/video annotation using hashing, graph learning and deep learning techniques.
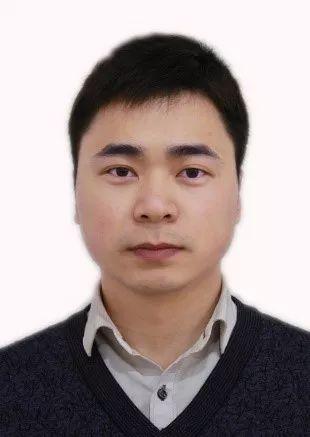
报告题目:Hierarchical LSTM with Adjusted Temporal Attention for Video Captioning
摘要:Recent progress has been made in using attention based encoder-decoder framework for video captioning. However, most existing decoders apply the attention mechanism to every generated word including both visual words (e.g., “gun” and “shooting”) and non-visual words (e.g. “the”, “a”). However, these non-visual words can be easily predicted using natural language model without considering visual signals or attention. Imposing attention mechanism on non-visual words could mislead and decrease the overall performance of video captioning. To address this issue, we propose a hierarchical LSTM with adjusted temporal attention (hLSTMat) approach for video captioning. Specifically, the proposed framework utilizes the temporal attention for selecting specific frames to predict the related words, while the adjusted temporal attention is for deciding whether to depend on the visual information or the language context information. Also, a hierarchical LSTMs is designed to simultaneously consider both low-level visual information and high-level language context information to support the video caption generation. To demonstrate the effectiveness of our proposed framework, we test our method on two prevalent datasets: MSVD and MSR-VTT, and experimental results show that our approach outperforms the state-of-the-art methods on both two datasets.
朱磊
简介:博士,昆士兰大学博士后研究员,研究方向为多媒体内容分析与检索。2015年获得华中科技大学计算机博士学位。2015年7月至2016年7月在新加坡管理大学担任博士后研究员。目前以第一作者身份在MM, AAAI, IJCAI,IEEE Transactions on Knowledge and Data Engineering, IEEE Transactions on Multimedia, IEEE Transactions on Cybernetics等顶级国际会议和国际期刊上发表论文30余篇,并担任多个顶级国际期刊和国际会议的审稿人。
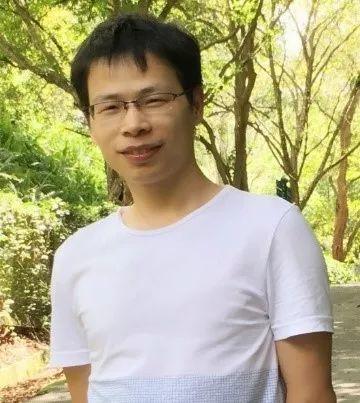
报告题目:Exploring Consistent Preferences: Discrete Hashing with Pair-Exemplar for Scalable Landmark Search
摘要:Content-based visual landmark search (CBVLS) enjoys great importance in many practical applications. In this paper, we propose a novel discrete hashing with pair-exemplar (DHPE) to support scalable and efficient large-scale CBVLS. Our approach mainly solves two essential problems in scalable landmark hashing: 1) Intra-landmark visual diversity, and 2) Discrete optimization of hashing codes. Motivated by the characteristic of landmark, we explore the consistent preferences of tourists on landmark as pair-exemplars for scalable discrete hashing learning. In this paper, a pair-exemplar is comprised of a canonical view and the corresponding representative tags. Canonical view captures the key visual component of landmarks, and representative tags potentially involve landmark-specific semantics that can cope with the visual variations of intra-landmark. Based on pair-exemplars, a unified hashing learning framework is formulated to integrate visual preserving with exemplar graph and the semantic guidance from representative tags. Further, to guarantee direct semantic transfer for hashing codes and remove information redundancy, we design a novel optimization method based on augmented Lagrangian multiplier to explicitly deal with the discrete constraint, the bit-uncorrelated constraint and balance constraint. The whole learning process has linear computation complexity and enjoys desirable scalability. Experiments demonstrate the superior performance of DHPE compared with state-of-the-art methods.
毛先领
简介:北京大学理学博士,IEEE TIST 和EMNLP等国际期刊会议评审人。已在AAAI,IJCAI, TKDE, CIKM, EMNLP, COLING等国际期刊会议上发表10余篇论文。主要研究机器学习与网络数据挖掘,具体研究Topic Modeling和Learn to Hashing等。所指导学生已在百度、中科院信工所、英国爱丁堡大学等IT公司或科研院所就业或者继续深造。
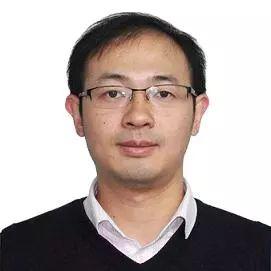
报告题目:Similarity-preserved Hashing Schemas for Probability Distributions
摘要:To compare the similarity of probability distributions, the information-theoretically motivated metrics like Kullback-Leibler divergence (KL) and Jensen-Shannon divergence (JSD) are often more reasonable compared with metrics for vectors like Euclidean and angular distance. However, existing similarity-preserved hashing algorithms cannot support the information-theoretically motivated metrics for probability distributions. In this talk, we will introduce several novel hashing schemes for approximate nearest neighbors search in high-dimensional probability distributions. Furthermore, extensive empirical evaluations well illustrate the effectiveness of the proposed hashing schemas on six public image datasets and two text datasets, in terms of mean Average Precision, Precision@N and Precision-Recall curve.
孟雷
简介:现任Research Fellow,就职于新加坡南洋理工大学百合卓越联合研究中心(Joint NTU-UBC Research Center of Excellence in Active Living for the Elderly —LILY)。目前研究重点聚焦在无监督学习和深度学习算法,以及面向老年健康的人工智能与大数据分析问题。担任并参与包括基于照片的老年饮食管理推荐、老年电商平台以及智能家居等三个项目的管理和算法研发。在国内外著名期刊和会议例如TKDE、 TNNLS、 SDM、 ICMR以及 IJCNN上发表学术论文十余篇,担任Applied Soft Computing期刊的编委,担任IWPR以及ICCSE会议的程序或技术委员会成员, 担任KDD、ICDM、SDM、TNNLS以及Applied Intelligence等会议和期刊的审稿人,是国际神经网络协会(INNS)和电子与电气工程师协会(IEEE)的会员
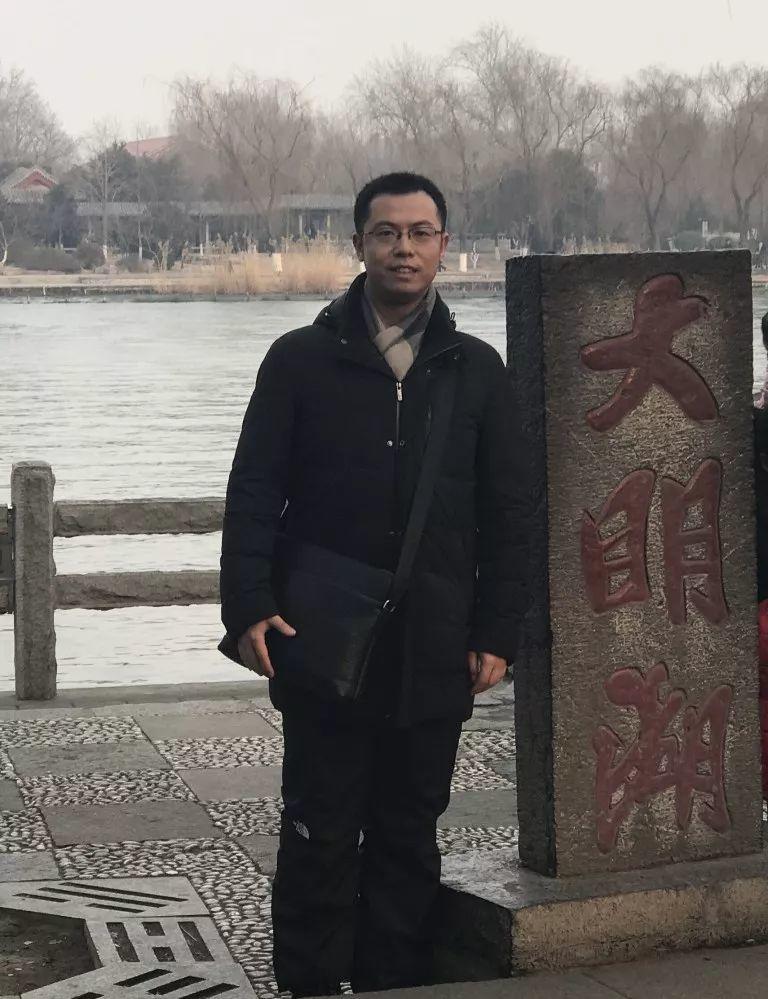
报告题目:Recent Advances in Estimating Air Quality from Single Photo of Daily Living
摘要: The issue of particulate matter in the air is a global question, challenging the health of people, especially children and the aging population. Thus, knowing the quality of air around them becomes an important step for daily activity arrangement. Besides purchasing and taking sensors, a more desired way is to achieve this by taking a photo using own phone. This talk will elaborate recent advances in air quality evaluation using different photo sources, illustrate technical challenges in doing so using phone photos of daily living, and present our solutions based on a novel haze region segmentation method and a cascaded CNN variant handling haze/non-haze classification and incorporating prior knowledge on the degree-progression nature of target classes in haze level prediction.
韩亚洪
简介:天津大学计算机学院教授、博士生导师,研究方向为多媒体分析、计算机视觉和机器学习。2012年3月博士毕业于浙江大学计算机学院,博士毕业被天津大学直接聘为副教授。是CCF优秀博士学位论文奖获得者(2012年度)、教育部新世纪优秀人才支持计划入选者(2013年度)。在国家留学基金委资助下,于2014年11月至2015年11月在加州大学伯克利分校做访问学者。2016年在天津大学破格晋升正教授。是《Frontiers of Computer Science》的青年编委和《Neurocomputing》、《World Wide Web Journal》、《Journal of Visual Communication and Image Representation》等期刊的客座编辑;是CCF高级会员,曾任YOCSEF天津副主席(2014年度),是ACM SIGMM China Chapter委员。近年来,在国内外重要期刊/会议发表论文60多篇,主持多项国家自然科学基金和天津市应用基础研究项目,作为学术骨干或课题负责人分别参与973、国家自然科学基金重点和国家社科重大等项目。
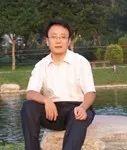
报告题目:序列深度学习的视频分析技术
摘要:近年来,以卷积神经网络(CNNs)为代表的深度特征学习方法在图像和视频分析中得到广泛应用,并在最近的大规模图像分类(如:ILSVRC)和视频动作识别(如:THUMOS)等任务中取得了最优性能。本报告围绕序列深度学习方法在视频分析中的应用展开,首先介绍视频分析中的深度时序注意力模型和方法,并展示其在视频分析和识别任务上的良好性能;视频的视觉内容相对于图像更加复杂,且在时空邻域上的依赖关系难以刻画,已有相关研究在视频深度特征学习上取得了进展(如TDD和TwoStream),本报告将介绍在深度时序量化方法上的最新研究进展,并讨论其在视频动作识别和视频语义描述生成中的应用。
何苯
简介:博士, 副教授,硕士生导师。2010年8月起,就职于中国科学院大学 计算机与控制工程学院。研究方向包括信息检索、自然语言处理。曾担任 ACM SIGIR, ACM CIKM, ECIR, AIRS 等国际学术会议的程序委员会委员,并为 ACM TOIS, JASIST, IP&M 等期刊审稿。在相关领域的国际会议和期刊发表了50多篇论文和著作。曾主持国家自然科学基金项目两项,北京市自然科学基金项目一项。
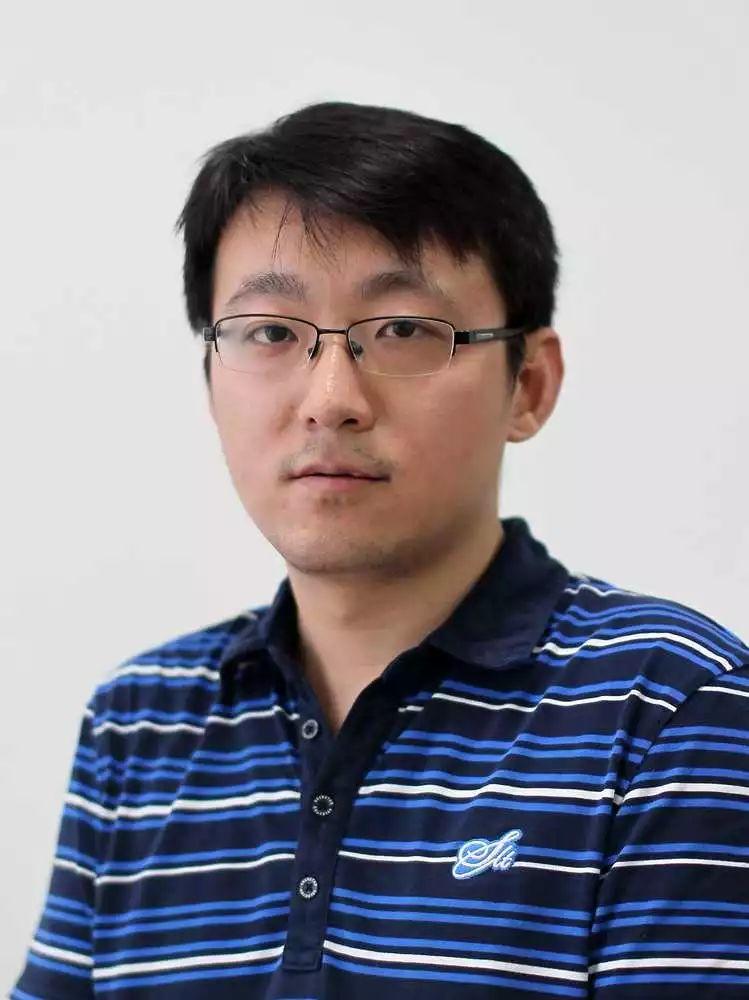
报告题目:词嵌入在信息检索中的应用
摘要:词/句嵌入方法通过考虑词/句的上下文信息建立词/句的分布式语义向量,在自然语言处理领域已成功得到广泛应用。此外,传统的信息检索模型在计算文档相关性时主要基于词项的精确匹配,并未考虑到查询和文档间的语义关联,语义信息的引入可望进一步提高模型的检索效果,因而近年来,词/句嵌入在信息检索中的应用成为了一个研究热点。本讲拟以综述的方式介绍词/句嵌入在信息检索中的应用概况。
张伟男
简介:1985年出生,哈尔滨工业大学计算机学院社会计算与信息检索研究中心。2014年7月在哈尔滨工业大学计算机科学与技术学科获得工学博士学位。研究兴趣包括聊天机器人、自然语言处理、信息检索。目前为中国中文信息学会信息检索专委会委员,中国人工智能学会青年工作委员会委员。曾获黑龙江省科技进步一等奖、中国人工智能学会“合创杯”第二届全国青年创新创业大赛三等奖、首届“百度奖学金”、哈尔滨工业大学第六届研究生“十佳英才”荣誉称号、第十九届全国信息检索学术会议优秀学生论文奖。
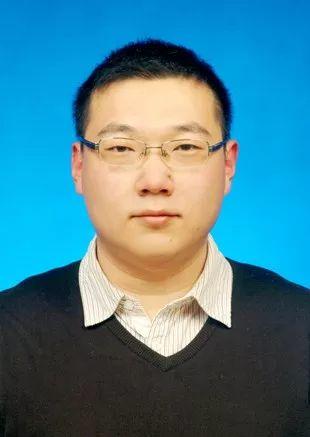
报告题目:开放域对话生成研究
摘要:近年来,人机对话技术受到了学术界和产业界的广泛关注。学术上,人机对话是人机交互最自然的方式之一,其发展影响及推动着语音识别与合成、自然语言理解、对话管理以及自然语言生成等研究的进展;产业上,众多产业界巨头相继推出了人机对话技术相关产品,如个人事务助理、虚拟情感陪护机器人、娱乐型聊天机器人等等,并将人机对话技术作为其公司的重点研发方向。以上极大地推动了人机对话技术在学术界和产业界的发展。本次报告我们主要介绍开放域对话生成的前沿研究工作,同时结合具体的人机对话系统介绍开放域对话生成的应用。
李旭涛
简介:Xutao Li is now an associate professor in Harbin Institute of Technology, China. Prior to this, he was a postdoc research fellow at the School of Computer Engineering, Nanyang Technological University (NTU). He received the PhD and Master in Computer Science from Shenzhen Graduate School, Harbin Institute of Technology in 2013 and 2009, and the Bachelor from Lanzhou University of Technology in 2007. His research interests include data mining, machine learning and social network analysis. He has published more than 30 papers, including TKDE, TNNLS, TIP, SIGKDD, SIGIR, WWW, AAAI, IJCAI, ICDE, CIKM, etc.
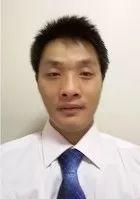
报告题目:Ranking Methods with Applications to Mining Heterogeneous Networks: Centrality Analysis and Recommendation
摘要:In this talk, I will present three of my recent work for mining heterogeneous networks. All the three work are ranking based methods, which can be applied to centrality analysis and recommendation tasks in heterogeneous networks. In the first part, I introduce the MultiRank model, which extends the conventional PageRank into multiplex networks, by proposing a tensor product-based Markov chain. The stationary distribution of the Markov-chain, corresponding to the z-eigenvector of a stochastic tensor, can reflect the importance of nodes and edges at the same time. In the second part, I introduce the HeterRS method, which can solve the recommendation problem in heterogeneous network (e.g., EBSN), by proposing a multivariate Markov chain with automated parameter learning. Finally, I will give a brief presentation for the Rank-GeoFM model, which learns a ranking based factorization model for POI recommendation. In each part, experimental results will be shown to verify the effectiveness of the proposed models.
组织委员会
论坛主席: 马军 (山东大学) 尹义龙 (山东大学)
组委会主席: 聂礼强 (山东大学) 崔超然 (山东财经大学) 聂秀山 (山东财经大学) 陈竹敏 (山东大学)
组委会委员: 张春云 (山东财经大学) 甘甜 (山东大学) 宋雪萌 (山东大学) 刘 倩 (山东大学)